Autonomous quality control systems are essential in modern manufacturing and offer efficient, accurate, and cost-effective alternatives to manual inspections. These systems use advanced technologies to ensure product quality and improve both reliability and efficiency. The shift from manual to automated quality control inspections marks a significant advance in quality control, driven by the need for more reliable and efficient processes in highly productive manufacturing environments. The increasing use of industrial artificial intelligence has further accelerated this process. Automation in quality control directly translates into improved product quality as systems perform detailed inspections more precisely and quickly than manual methods. This not only improves the quality of the final product but also reduces the likelihood of defects and recalls.
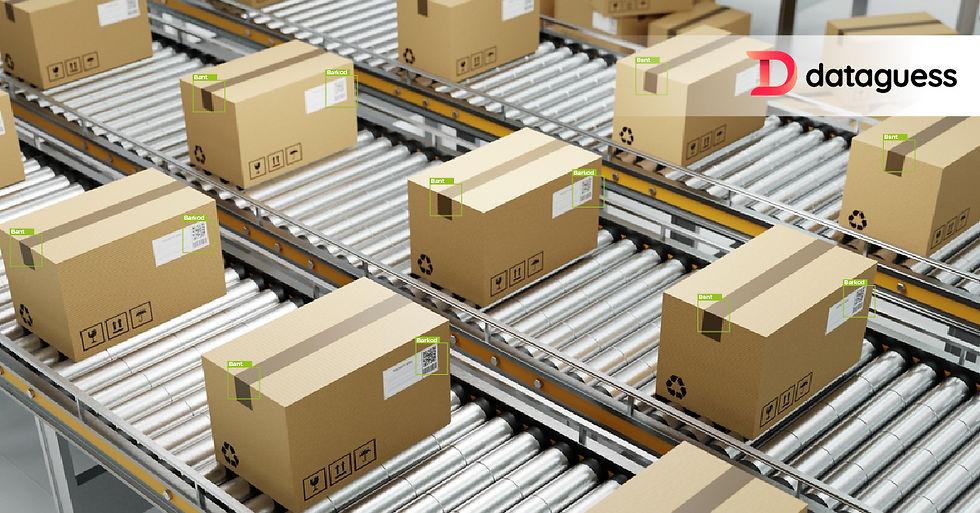
Brief History and Development of Autonomous Quality Control Systems
Quality control has evolved significantly from its origins in medieval Europe, where artisan guilds set the first standards. The Industrial Revolution ushered in the factory system, which shifted the focus from craftsmanship to mass production and emphasized product inspection. The late 19th and early 20th centuries saw further developments with the introduction of the Taylor System and statistical quality control (SQC) techniques in the United States. Japan's emphasis on quality after World War II led to the development of Total Quality Management (TQM), which focuses on improving all organizational processes. Today, the quality movement includes advanced technologies such as artificial intelligence and marks a significant evolution in autonomous quality control systems.
Transition from Manual Quality Control to Autonomous Quality Control
The shift from manual quality control inspections to autonomous quality control inspections marks a significant advance in manufacturing processes. This shift is driven by the need for more reliable, efficient, and consistent quality control in high-throughput production environments. With the ability to process large volumes of products quickly and accurately, autonomous systems are becoming an invaluable tool for manufacturers aiming to maintain high quality standards while reducing costs and preventing costly errors and rework.
Comparison of Manual and Autonomous Quality Control Inspections
Traditional Manual Visual Quality Control Systems
Process: Qualified inspectors manually evaluate each product to identify defects and deviations from quality standards.
Challenges: The biggest drawback is the possibility of human error. Even well-trained inspectors are prone to making mistakes, especially when inspecting large volumes of products. In addition, manual auditing can produce variable results due to the different techniques used by various auditors, which can lead to inconsistency.
Cost and Time: Manual inspection is labor-intensive and time-consuming, slowing down the production process and potentially leading to higher costs and lower production quality.
Autonomous Quality Control Systems
Technology: Automated Optical Inspection (AOI) uses advanced imaging methods, high-resolution cameras, and artificial intelligence algorithms to automatically detect defects and deviations from set quality standards.
Process: AOI systems highlight discrepancies or anomalies by comparing images of products taken during production against a reference image.
Advantages: AOI systems offer increased speed and efficiency, improved accuracy and consistency, improved production quality control, cost effectiveness, and a significant reduction in human error.
Industry 4.0 and Autonomous Quality Control
The rise of Industry 4.0 plays a critical role in the evolution of autonomous quality control systems. This transformation is characterized by the digitalization of production processes and the integration of smart technologies. As one of the cornerstones of Industry 4.0, autonomous quality control has an important place in improving the efficiency of production lines and product quality.
Image Processing Technologies and Autonomous Quality Control
Advanced image processing technologies are key components of autonomous quality control systems. By analyzing high-resolution images of products, these technologies quickly and accurately detect defects and deviations. Image processing significantly increases the precision and speed of quality control, especially in complex manufacturing processes. Today, quality control with industrial artificial intelligence is rapidly gaining ground in the manufacturing industry.
Artificial Intelligence and the Future of Autonomous Quality Control
Artificial intelligence plays a central role in the future of autonomous quality control systems. By analyzing complex datasets, AI algorithms make quality control processes more intelligent and predictable. This technology significantly increases the efficiency and reliability of production processes, especially in predictive maintenance and fault detection.
Use of Artificial Intelligence in Manufacturing and Autonomous Quality Control Application Examples
As Dataguess, let's talk about one of our artificial intelligence application examples in production. Below is the work we did with the manufacturer company for the quality control problems of automobile console buttons:
Problem: We detected the color difference between the buttons, whether the buttons are in the right slot or not, and the presence-absence controls of the buttons on the assembly line with our camera solution integrated with our industrial artificial intelligence solution.
Solution: We detected color differences within the tolerance range specified by the company and analyzed them by collecting images. With Dataguess Inspector, we checked whether the buttons were in the correct slot, performed presence-absence checks, and labeled the problems belonging to the case (previously detected) to be captured on the resulting dataset. Data enrichment, artificial intelligence model improvements, tests, and integrations were made, the trained artificial intelligence model was deployed, and live monitoring started.
Result: After taking the system live, we ensured that caught cases were reported to the operator via siren, buzzer, or monitor so that the system could be stopped or necessary actions could be decided.
Challenges and Limitations of Autonomous Quality Control
While autonomous systems offer many advantages, they also face challenges. Implementation costs, the need for specialized training, and integration with existing production processes can be significant obstacles. In addition, over-reliance on automated systems can lead to a lack of skills among quality control personnel.
Comments